Meeting technology and methodology into Health Big Data Analytics scenarios
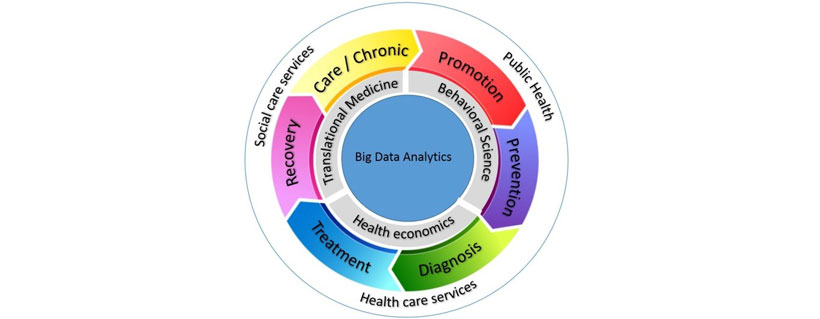
Health organizations are collecting more data from a wider array of sources at greater speed every day. The analysis of this vast amount and array of data creates new opportunities to deliver modern personalized health and social care services.
Healthcare analytics provide methods and processes for extracting and transforming raw medical data into meaningful insight, new discovery and knowledge that supports efficient and effective healthcare decisions. However, an agile methodological approach driving the full process pipeline, from raw data to effective knowledge activation in the medical daily routines, is still missing. Our approach focuses the classical health areas and activities combining 1) new technological paradigms developed in the arena of analytics and big data and 2) novel methodological approaches from translational medicine, health economics and behavioral sciences. The main objective of applying health data analytics and big data technologies together with other branches of knowledge such as social and behavioral sciences is to develop an innovative analytical framework that contributes to the improvement across the whole health continuum (promotion, prevention, diagnosis, treatment, recovery and care/chronic).
Health organizations face a new scenario where analytical tools must accommodate both, traditional business intelligence and novel big data analytics approaches, resulting in important technological and methodological challenges to be tackled. These challenges are the force that drives the design of the analytical framework under the viewport of the methodology high lined in the previous figure. From this perspective instead defining what big data is in terms of V’s, we propose to figure out it in terms of technological solutions harnessing new business requirements, as 1) NoSQL (Not Only SQL) databases, 2) distributed storage and distributed computing 3) Distributed machine learning and 4) Virtualization technologies in their different degrees (Hypervisors, Linux containers and application containers).
The analytical framework supporting the UOC-BSA chair analytical objectives, structures different solutions and approaches in the four previous lines, into four-layer logical platform levels, 1) The source and storage layer, 2) The data layer, 3) The cognitive layer and 4) the metadata layer, as well as the connectors and data transfer solutions between layers.
In view of the different analytical requirements of each specific analytical project, the technological platform to be implemented should be easily adaptable to different scenarios, covering both a more traditional analytical approach and more innovative environments where technologies associated with Big Data Analytics are needed. In this context, several decisions should be made with respect to the data storage approach, distributed design, tool selection and analytics models. To this end, we considered a set of design guidelines driving the technical and structural decisions about the construction of the framework (heterogeneous data support, agility and flexibility, metadata and data governance support, solution stability, use of standards and so on).
This way we developed the methodological approach, the technical structure and the design guidelines needed to deploy the data lab supporting the analytical projects developed in the context of the chair.